Hands-On Machine Learning with Scikit-Learn, Keras, and TensorFlow: Concepts, Tools, and Techniques to Build Intelligent Systems 2nd Edition
Giới thiệu sách
Through a series of recent breakthroughs, deep learning has boosted the entire field of machine learning. Now, even programmers who know close to nothing about this technology can use simple, efficient tools to implement programs capable of learning from data. This practical book shows you how.
By using concrete examples, minimal theory, and two production-ready Python frameworks—Scikit-Learn and Tensor Flow—author Aurélien Géron helps you gain an intuitive understanding of the concepts and tools for building intelligent systems. You’ll learn a range of techniques, starting with simple linear regression and progressing to deep neural networks. With exercises in each chapter to help you apply what you’ve learned, all you need is programming experience to get started.
- Explore the machine learning landscape, particularly neural nets
- Use Scikit-Learn to track an example machine-learning project end-to-end
- Explore several training models, including support vector machines, decision trees, random forests, and ensemble methods
- Use the Tensor Flow library to build and train neural nets
- Dive into neural net architectures, including convolutional nets, recurrent nets, and deep reinforcement learning
- Learn techniques for training and scaling deep neural nets.
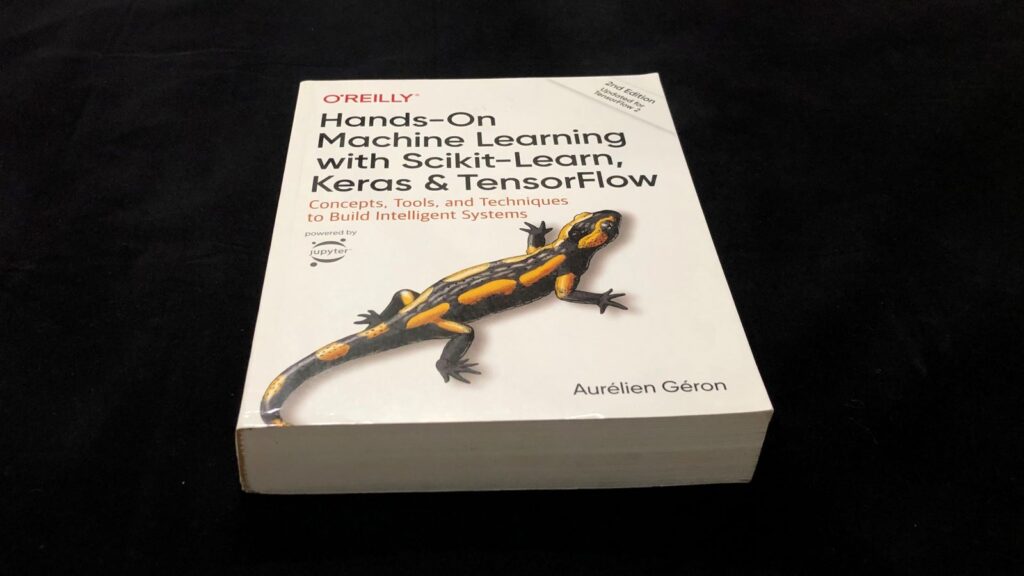
- Sách đang bán: $61.26 (1,420,000 ₫)
- Condition: New
- Amazon: https://amzn.to/2ynP3Dt
- Youtube: https://youtu.be/PtVHycdg8nk
This book gives you a hands-on approach to learning by doing. As opposed to the trendy deep learning books that dive deep into the weeds from the start, this book starts with the more traditional ML approaches (the Scikit-learn part) giving you a great deal of context and practical tools for solving all kinds of problems. Only after does he transition into deep learning concepts, giving you both a great overview and the background to understand when and where to apply the various techniques. Its code-focused so you’ll have the option to run working code on real problems throughout the book.
Most important for me, he focuses on explanation over hand-wavy equations that are rampant in other ML books. I say hand-wavy because they typically go like so: “Here’s a hard concept. Rather than explain it well, I’ll give you some linear algebra and calculus equations, remind you that this is stuff you should have learned in high school, and then move on.” Authors probably feel justified in doing this, but after reading a book like this you understand what they are really doing: Skipping the hard-part of breaking difficult concepts down into chunks that can be consumed by a competent programmer, who is perhaps not an expert in “high school” math. Moreover, this author does so without dumbing down the content. That’s the mark of someone who well understands both the content and the audience.
This book is long and dense, and serves as both a guide and a reference. It is not a quick read / overview or light reading type book.